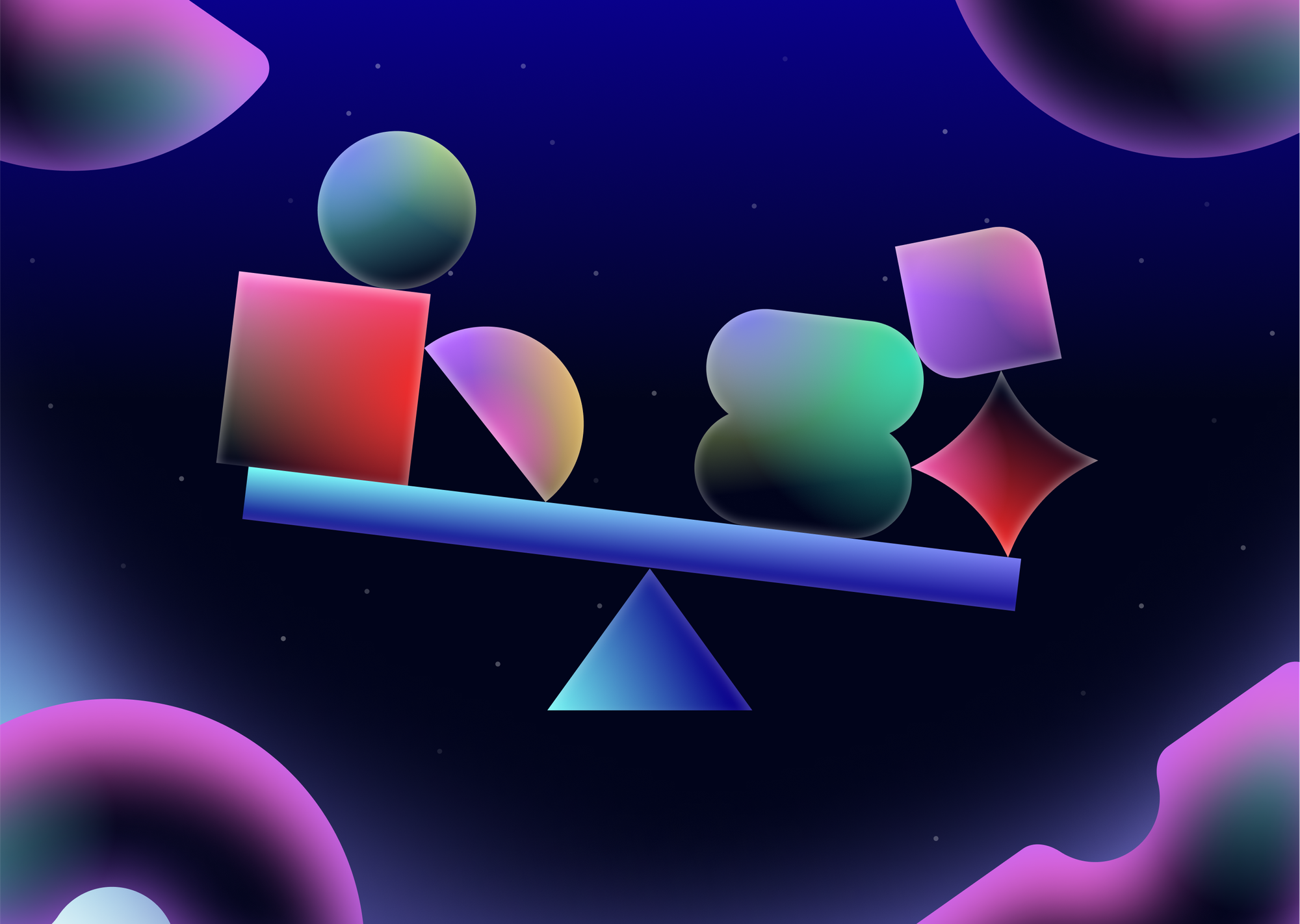
We’ve all seen Generative Artificial Intelligence (GenAI) pushed as the “solution” to every problem. AI can make things faster, but does it really make things better? And more importantly, does it replace the need for human QA work? The latest research has an answer: No, it doesn’t — but it does change how QA works.
GenAI is reshaping how QA professionals approach testing with its ability to process massive datasets, identify patterns, and execute tasks quickly. However, integrating GenAI is not a straightforward journey. While GenAI opens doors to innovation and new approaches in testing, it also presents challenges, such as social impacts, technical complexities, and ethical dilemmas.
We don’t just test software — we experience it. This perspective defines the value testers bring to the table. By stepping into users’ shoes, we uncover insights, ask critical questions machines cannot, and tackle complex scenarios creatively and empathetically. GenAI is not a magic solution — it’s an enabler that can be used strategically to enhance the QA process.
Testers must learn how to leverage AI to stay relevant in this evolving industry. However, it’s equally important for testers to apply their unique human strengths like intuition, critical thinking, and contextual understanding. Striking that balance starts with understanding various aspects of AI so we can learn how to navigate this transformation effectively.
Social aspects of GenAI integration
GenAI can have a dangerous influence on how we speak
AI systems influence how people communicate, especially as tools like chatbots and voice assistants become part of everyday life at home and in the office. One recent study [1] suggests that humans increasingly imitate large language models (LLMs) in their spoken and written language. The study also highlights GenAI's potential to unintentionally reduce linguistic diversity or be deliberately misused for mass manipulation.
In testing, this could mean a loss of details because GenAI processes information in generalized terms. It’s also important to remember that if training data is biased — and it often is — using GenAI can reinforce stereotypes and introduce more bias into testing.
AI affects our willingness to share knowledge
Another study [2] revealed that ChatGPT has led to a 25% drop in activity on Stack Overflow, a key reference website where programmers share knowledge and solve problems. This substitution threatens the future of the open web, as interactions with GenAI models are not added to the shared pool of online knowledge.
Moreover, this phenomenon could weaken the quality of training data for future models, as machine-generated content cannot fully replace human creativity and insight. This shift might make it harder for us as QA professionals to investigate problems effectively and get information from independent sources.
Relying on these tools instead of engaging directly with colleagues can complicate the testing process. While AI-driven platforms enable faster idea exchanges, they may inadvertently discourage genuine collaboration.
Mitigating risks associated with the social impacts of AI
To effectively address these social risks, organizations should implement proactive strategies targeting challenges at multiple levels. The key is to foster ongoing live communication while driving technological innovation forward.
Company level
1. Training programs for testers and quality engineers
Identify unique needs: Training tailored to address specific challenges that testers and quality engineers face in AI applications opens doors for new QA opportunities. For instance, at Regent AB, we do a series of AI training sessions. These programs include hands-on sessions that focus on understanding how LLMs work and evaluating AI algorithms for fairness and biases.
We also host workshops that emphasize ethical considerations in AI, particularly regarding the social risks that may emerge during testing. This makes our team members more attractive on the job market and equips them with up-to-date AI engineering skills.
Continuous learning opportunities: Keep training resources up to date with the latest in AI testing methodologies. For example, we do our sessions bi-weekly to address any emerging questions and secure testing resources for any customer requests.
Cross-disciplinary learning: Incorporating knowledge from sociology, journalism, and ethics into training programs can help testers and software engineers appreciate the broader social implications of their work, enabling them to design and test AI systems with greater responsibility. One example of this could be a cross-cultural seminar where testers and engineers with different backgrounds discuss their unique perspectives on AI fairness, such as addressing language biases in user interfaces or cultural nuances in data interpretation, creating a deeper understanding of global impacts.
2. Inclusive test design practices
Diverse teams: Assembling diverse software and testing teams is essential for ensuring a variety of perspectives. Thanks to their varied backgrounds and perspectives, diverse teams are more effective at identifying risks and potential biases in AI applications early in the process, uncovering issues that might otherwise go unnoticed.
Stakeholder engagement: Actively involving stakeholders, including end users, during the development and testing phases fosters inclusivity. Understanding user perspective is more important than ever, as only humans can fully grasp the nuances of human emotions, behaviors, and contexts. For instance, while an AI application might analyze usage patterns, only a human tester can empathize with the frustration of navigating a confusing interface or recognize how cultural differences might impact the interpretation of AI-driven recommendations.
Team level
1. Balancing use of GenAI and human expertise
GenAI can be used to maximize the strengths of repetitive tasks. LLMs shine at language processing and pattern detection. Use it as a starting point to find what fits your project best.
A recent example in one of our projects was log scanning, where LLMs are used to detect anomalies in large volumes of logs. This way, the team eliminates exhaustive work, allowing human testers to focus on nuanced evaluations.
Another case is the generation of the first draft of documentation. Using the power of AI, it becomes easier to overcome the initial friction of starting from scratch.
2. Enhancing team collaboration
Workshops are an excellent opportunity for testers and developers to collaborate, discuss challenges, and find solutions. Workshops also help create a culture of knowledge sharing within the team. By encouraging testers to share their experiences and techniques, we enhance the entire team’s expertise.
3. Celebrating small wins
Recognizing testers' achievements, whether during team meetings or in newsletters, creates a sense of appreciation. This approach extends to sharing insights about GenAI applications. Our team members actively support one another by regularly exchanging ideas and demonstrating useful GenAI use cases. For one of our client’s projects, we have an AI "fika," which is a Swedish tradition of connecting over coffee and cinnamon buns. This relaxed setting fosters open conversations about AI challenges and ideas, making it easier to share knowledge and build stronger team connections.
Individual level
1. Don’t skip real-world communication
To use GenAI intelligently, testers must understand that it might be tempting to skip real-world conversations and ask GenAI for answers instead. However, this can lead to miscommunication and a lack of shared understanding among team members, which is essential for effective problem-solving. While AI-driven platforms enable faster idea exchanges, they may inadvertently discourage genuine collaboration.
Handling repetitive tasks such as generating test data, analyzing large datasets, or spotting patterns in results is a good example of GenAI synergy with human expertise. It’s essential to follow up by validating these outputs through collaboration and human judgment to ensure they align with project goals and maintain fairness.
Another example of maintaining communication while using the latest tech is using AI tools to gather data or identify patterns and then sharing these findings in team meetings. For example, after using AI to analyze test logs, testers can present their conclusions and invite feedback.
2. Stay relevant
On the other hand, staying relevant in the job market requires individual test engineers to actively engage with emerging trends and the latest AI developments. While it’s not essential to master every aspect of AI, understanding its foundational concepts and industry impact is important. To incorporate AI into your workflow effectively, start using AI tools to automate small, repetitive tasks. You should also attend AI-focused workshops, webinars, or certifications to build practical skills and keep up-to-date with advancements.
Additionally, explore how AI can complement your current testing strategies, such as identifying edge cases or improving defect detection. This understanding boosts job security and elevates your value in the market by demonstrating adaptability, innovation, and informed decision-making. Remember, AI is here to stay, and embracing it thoughtfully is the key to thriving in tech.
Technical aspects of GenAI integration
GenAI models thrive on large volumes of high-quality data, yet there is a growing challenge of insufficient data availability [4].
The consent crisis limits data access
The consent crisis has emerged as a significant challenge in the field of GenAI, directly impacting the availability of high-quality data for training purposes. As data privacy regulations tighten globally (GDPR in Europe, CCPA in the US), organizations face increasingly tightened requirements to collect, store, and process user data.
This shift has led to substantial barriers in GenAI training. Users are becoming more reluctant to share personal information due to heightened awareness of privacy risks and a growing mistrust in corporate data practices. As a result, companies find themselves constrained, with limited access to the diverse, representative datasets needed to refine and train advanced AI models.
QA engineers must check what type of data is sent to AI tools and how it is handled. A common case is that we need to ensure data is anonymized correctly in AI applications. If anonymization methods are insufficient, sensitive user information might be exposed, causing a breach of privacy.
Ultimately, the consent crisis underscores the delicate balance between advancing GenAI capabilities and respecting individual privacy rights. QA teams need to be aware of data that has been used to train models used in the latest AI testing tools. If this data is used without proper consent, testers might unknowingly validate unethical AI outputs, leading to reputational, compliance, and legal risks for the organization.
Synthetic data and self-consuming models
Synthetic data has emerged as a potential solution to data availability and privacy challenges because it is not tied to real user information. However, synthetic data introduces its own risks and challenges.
One of the primary concerns is the phenomenon of "model collapse." This occurs when models trained exclusively on synthetic data are iterating on their own errors and biases [6] [7]. Unlike real-world data, which inherently contains diversity and unpredictability, synthetic data reflects the limitations and assumptions of the models that generate it. Over time, training on such data creates a feedback loop where errors and biases are amplified.
Another issue is the loss of data diversity. Real-world datasets contain a wide range of nuanced, context-rich patterns. Synthetic data, on the other hand, lacks this complexity and can result in models that fail to capture the full spectrum of potential inputs, reducing their accuracy and adaptability.
Synthetic data can have significant implications in testing applications across fields like healthcare, finance, or autonomous systems. It often fails to capture the full complexity of real-world scenarios, leading to gaps in test coverage and accuracy.
In healthcare testing, for instance, synthetic data might fail to capture rare conditions or the nuanced interactions between medical variables, leading to gaps in test coverage for diagnostic tools or treatments. This issue arises because, statistically, there are fewer ill people compared to relatively healthy individuals, resulting in insufficient real-world data to generate high-quality synthetic data for comprehensive testing scenarios.
Similarly, in autonomous systems testing, synthetic data often struggles to replicate the unpredictable behaviors seen in real-world scenarios, such as the variability of human decision-making in traffic. To address this, synthetic data should be combined with real-world datasets and subjected to strong validation processes, ensuring that testing scenarios reflect actual operating conditions.
Addressing technical limitations of GenAI in testing
Testing teams and QA engineers should start by understanding the core functionalities of GenAI tools and their specific use cases in testing. Start with manageable, low-risk tasks that allow teams to gain hands-on experience and build confidence with GenAI tools. For example, GenAI can be used to generate scripted test cases for simple workflows or analyze logs for recurring issues.
It’s important to clearly define the objectives of GenAI integration, such as accelerating regression testing, improving defect detection efficiency, or enhancing test coverage. Always consider its limitations, such as potential inaccuracies or inability to handle complex edge cases without human oversight. Here is a quick how-to guide to make the most out of GenAI to improve the testing process:
Collaborate with experts
Combine GenAI tools with the expertise of domain experts to ensure deep analysis and mitigate reasoning gaps. Involve subject matter experts during critical testing phases to validate AI-generated outputs, particularly when dealing with specialized fields such as healthcare, social insurance, transportation, or embedded systems.
For example, domain experts can help identify subtle biases or errors in AI predictions that might not be evident to testers alone. Encourage regular knowledge-sharing sessions between testers and experts to align objectives and improve the overall quality of testing. Additionally, create a feedback loop where experts review and refine AI-driven test scenarios, ensuring they are realistic and relevant to real-world use cases.
An example would be involving psychologists and healthcare professionals to assess a suicide prevention system in public transport by collaborating directly with QA professionals during system validation. These experts can guide testers in designing test scenarios that evaluate how the AI system detects behavioral patterns and triggers interventions, such as identifying unusual body language or prolonged stationary behavior. They can also help testers interpret false positives or false negatives in AI predictions, providing critical insights to refine test cases.
Do not blindly trust GenAI output
This is a principle that directly applies to testing systems using AI-generated content. Evaluating Generative AI outputs is critical, particularly when these systems are used in real-world applications. Blindly trusting the output of GenAI can lead to significant issues, especially in edge cases where the input data is sparse, ambiguous, or unique. As testers, ensuring accuracy, relevance, and reliability in AI-generated outputs requires a thorough approach.
Clear acceptance criteria must be formed to define what constitutes a correct or usable output. Automated testing frameworks can help verify that generated outputs meet these criteria, while an exploratory review of a representative sample ensures that any nuanced errors are caught. This combination of automated and exploratory-style testing helps maintain high standards of accuracy.
Relevance testing ensures that the content generated by the model aligns with the input context. By simulating real-world scenarios, testers can validate that the outputs make sense and are contextually appropriate. This is especially important in applications like chatbots, content generation, and decision-support systems, where mismatched or irrelevant outputs can disrupt user experiences.
Finally, feedback mechanisms should be in place to allow users to flag incorrect or irrelevant outputs. The system's performance must be tracked through metrics such as relevance and error rates. This ongoing evaluation helps prevent biases and unfair decisions while highlighting the need for human intervention when necessary. For instance, the absence of such monitoring in Sweden’s social insurance AI-supported decision-making mechanisms last year raised significant questions about the practices of the social insurance authority.
Invest in strong basics
While GenAI tools can assist everyone, their true potential lies in the context of human expertise. We must understand its strengths, limitations, and the areas where it adds value. For beginners, the efficiency gains may be minimal as they are still developing their testing skills and learning how to integrate GenAI tools into their workflows. However, for experienced testers who have a deep understanding of testing principles and can critically evaluate GenAI outputs, these tools can help make routine tasks smoother.
I believe that GenAI can serve as excellent tutors and conversational partners, provided you know how to frame your questions effectively and know what to ask for. To achieve this, foundational testing skills must first be acquired. In my teaching experience, students who immediately dive into using AI tools without first understanding the foundational concepts of the testing process tend to make slower progress during the course.
Ethical aspects of AI integration
Lack of clear benchmarking methods
The lack of standardized benchmarking methods in the field of GenAI is a significant challenge, complicating efforts to evaluate and compare models objectively. Unlike traditional machine learning tasks, where established benchmarks and datasets provide clear metrics for performance evaluation, GenAI lacks a universal framework for assessing model capabilities.
One of the primary challenges in testing and assessing GenAI systems lies in the diversity of their applications, which include text generation, image and video synthesis, code creation, and conversational agents. Each of these domains requires specific and tailored evaluation criteria to make sure that outputs meet their intended purpose and maintain quality. However, the lack of universally agreed-upon testing standards for these systems creates fragmented approaches and metrics that are difficult to compare.
For example, testing a conversational agent might focus on user engagement, coherence, and intent accuracy. Meanwhile, testing code generation systems might emphasize correctness, efficiency, and readability. This inconsistency complicates the creation of comprehensive testing strategies. Testers must adapt their methods to the unique requirements of each application domain while advocating for more standardized evaluation frameworks.
Security concerns
Ethical issues, such as bias and fairness, also tie into security. A biased system can produce harmful or unfair outputs, leading to reputational damage or legal problems. As GenAI becomes part of more applications, it brings new security challenges that need to be addressed to keep these systems safe and reliable.
One key concern is the risk of data leaks. GenAI systems often use large datasets to learn and make predictions; some of this data might include sensitive or private information. Testers must ensure the systems do not accidentally reveal this confidential information in their outputs.
Another major issue is the risk of adversarial attacks. In these attacks, someone gives the system inputs designed to confuse it, make it malfunction, or bypass security controls. Testing should include trying out tricky inputs to see how the system reacts and to find any weak spots.
When GenAI is added to applications, it may depend on third-party models or APIs, which have their own vulnerabilities. This creates a supply chain risk, where weaknesses in external components affect the whole system. Testing must include checks to ensure these dependencies are secure and do not put the system at risk. A strong starting point for securing APIs that use LLMs is to follow the OWASP Top 10 guidelines, which provide comprehensive best practices to address common security vulnerabilities.
Finally, keeping these systems secure is not a one-time task. New threats can arise over time, and vulnerabilities that were not obvious initially might become clear later. Regular monitoring and updates are essential to stay ahead of these risks. Automated testing tools and periodic reviews can help ensure that the system remains secure as threats evolve.
Mitigating risks of ethical impacts of AI
Establish clear benchmarks
Metrics should address what is most important for your organization, whether it is handling edge cases effectively, ensuring consistent performance under diverse input conditions, or meeting predefined acceptance criteria. Engaging stakeholders to define these priorities based on organizational needs and objectives is critical.
Additionally, benchmarks must be tailored to the specific domain in which GenAI is applied, such as conversational agents, code generation, or data synthesis, ensuring that testing outcomes align with real-world demands and constraints while directly supporting the organization’s goals.
Address bias transparently
Regularly audit GenAI tools used in testing and GenAI-powered applications to identify and mitigate biases that might distort test results or create unfair outcomes. Focus on areas such as demographic representation in datasets, edge cases that could reveal systemic bias, and potential disparities in test coverage. Questions to guide this process include:
- Does the dataset represent all relevant user groups equally?
- Are there patterns of unfair outputs for specific groups?
- What mechanisms are in place to detect and correct biases during runtime?
Resource utilization
GenAI systems require significant computational power, leading to high energy consumption. We can advocate for optimization strategies within the testing process, such as using smaller, specialized models where appropriate or scheduling resource-intensive tests during off-peak hours. By balancing performance needs with energy efficiency, we contribute to reducing the environmental impact of testing without compromising on quality.
Continuous oversight
Monitor real-world performance and unintended impacts by implementing continuous feedback loops. This involves gathering data from production environments to identify discrepancies, unanticipated failures, or biases that may arise. Use this information to adjust workflows and improve overall testing reliability and fairness.
While automated monitoring systems are essential for capturing real-time data and identifying patterns, human oversight is equally important to interpret nuanced issues, provide context-aware evaluations, and address ethical considerations.
Establishing practices like an ethical committee and a security champion network is a great start, but remember that these roles must be clearly defined and actively involved throughout the lifecycle of GenAI integration. An ethical committee should oversee the testing and deployment phases, making sure that decisions align with organizational values and fairness principles. Meanwhile, a security champion initiative could focus on identifying vulnerabilities, developing mitigation strategies, and fostering a culture of security within the organization.
These practices can be further enhanced by setting up regular review sessions, encouraging collaboration between testers and domain experts, and providing tools to track ethical and security compliance metrics over time. This structured approach helps to contribute meaningfully to building trustworthy and secure AI systems.
The overall impact of AI depends on how teams use it
AI in QA represents progress over perfection. By integrating GenAI thoughtfully into testing workflows, testers and organizations can foster a future where technology amplifies human capabilities. This collaboration can deliver better quality and enhanced innovation in software. It’s a journey where every action — from minor improvements to major breakthroughs — contributes to meaningful outcomes. Testers hold the potential to drive this transformation and shape the future of QA.
However, this journey with GenAI isn’t without challenges. It requires careful navigation of social, technical, and ethical dimensions to maximize its benefits while minimizing risks. By addressing these aspects head-on, organizations can take advantage of GenAI’s potential, creating a balance between human ingenuity and technological power.
For further reading feel free to explore studies mentioned in the article:
[1] https://arxiv.org/abs/2409.01754
[2] https://academic.oup.com/pnasnexus/article/3/9/pgae400/7754871
[3] https://www.hbs.edu/ris/Publication%20Files/25-023_d09f0b39-4d4b-4828-b13e-df5dce799de0.pdf
[4] https://arxiv.org/abs/2211.04325
[5] https://arxiv.org/pdf/2407.14933
[6] https://openreview.net/pdf?id=ShjMHfmPs0
[7] https://arxiv.org/html/2410.16713v1
[8] https://arxiv.org/abs/2410.05229
[9] https://www.bloodinthemachine.com/p/ai-is-revitalizing-the-fossil-fuels
[10] https://www.washingtonpost.com/technology/2024/03/10/big-tech-companies-ai-research/
[11] https://www.nature.com/articles/s41586-024-07856-5/figures/1
[12] https://www.amnesty.se/aktuellt/sverige-forsakringskassan-ai-system/